On the Benefits of Photogrammetry vs LiDAR, and Positioning Technology
Capturing accurate mobile mapping data is more than making a balanced choice between camera-based systems or LiDAR scanners. Both technologies rely on accurate positioning to create a good trajectory, which is the precise path followed during data collection. This article explains
- how to create a good trajectory for any mobile mapping system
- what are the differences between LiDAR and photogrammetry
- why camera-based systems will most likely meet all your mobile mapping accuracy requirements.
Breaking Down Mobile Mapping
A mobile mapping system is more than a set of cameras combined with an optional laser scanner. Understanding the different components and their functions makes for a better understanding of how you can acquire accurate spatial data.
Mobile Mapping System Components
Any mobile mapping system integrates different technologies to collect 3D spatial data. These typically include the following components:
- Positioning systems, including a GNSS system and an IMU (inertial measurement unit)
- Mapping sensors, such as 3D laser scanners (LiDAR) or cameras for capturing high-resolution imagery
- On-board data storage and processing units, and additional equipment such as control systems and communication systems
Mobile Mapping for Visual Inspections
The popularity of LiDAR in different industries has led to a discussion of whether photogrammetry or LiDAR is the best option for acquiring high-quality spatial data at scale. While this is a relevant and useful discussion, in many cases it is irrelevant as not every use case requires 3D data with millimeter accuracy. For GIS-based inspection workflows, high-end systems and associated investments are unnecessary.
This explains why more entry-level camera systems have entered the market. Whereas high-end systems such as LiDAR scanners meet a data demand for survey-grade, high-accuracy spatial data, visual inspection tasks require imagery where such accuracies are not needed. That doesn’t mean it’s impossible to obtain spatial data with survey-grade accuracies using camera-based mobile mapping systems. How this is done is covered later in this article. We’ll now discuss the importance of a good positioning system, which is a requirement for any mobile mapping system.

The Importance of Positioning Technology for Acquiring Mobile Mapping Data
What matters most for mobile mapping jobs is creating a good trajectory. Before mobile mapping data is collected, the precise path that the mobile mapping system follows during data collection needs to be determined. Such a trajectory is a prerequisite for accurately georeferencing the collected spatial data, whether this is LiDAR, imagery, or other sensor data.
Creating a good trajectory requires the use of both Global Navigation Satellite System (GNSS) and Inertial Measurement Unit (IMU) data. GNSS provides raw positioning data from different satellite constellations. An IMU measures the moving platform’s acceleration and angular velocity, which is useful for navigation and positioning for example when GNSS signals are weak or unavailable. A spatially accurate trajectory provides a guaranteed level of accuracy that allows you to overlay any kind of visual data on top of that when performing inspection tasks in the office.
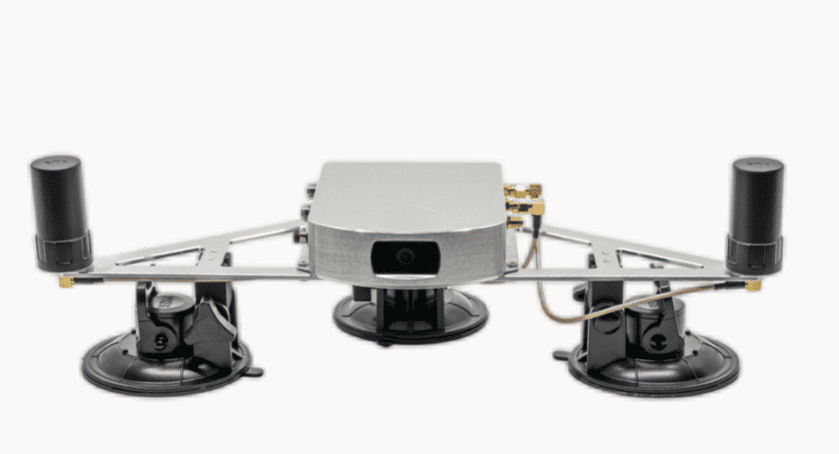
With added visual data such as LiDAR or imagery, it becomes possible to refine the accuracy a little more and do further alignment and analysis, including simultaneous localization and mapping (SLAM), or structure for motion (SfM). SLAM involves constructing or updating a map of an unknown environment while simultaneously keeping track of one’s location within it, while structure for motion is a photogrammetric range imaging technique for estimating three-dimensional structures from two-dimensional image sequences.
Comparing LiDAR vs Photogrammetry Accuracy
Now that we’ve discussed the value and importance of precise positioning systems for acquiring accurate mobile mapping data in the field, we’ll cover the differences between LiDAR and photogrammetry. Both are spatial data acquisition technologies that are in high demand in different industries that rely on current and accurate spatial data. After discussing the differences between the two, we’ll look at how both technologies can complement each other and how valuable information is derived from 3D point clouds.
Active versus Passive Sensors
LiDAR and photogrammetry are different technologies. LiDAR pulses are active sensors, which means they don’t need an additional light source to function. This means that it is possible to use them in the dark, whereas using a camera, you would need an additional light source.
Point Clouds and Imagery Represent the Environment Differently
The imagery from photogrammetric systems is easier to interpret than LiDAR data as they represent the surroundings in their natural colors as perceived by humans. This becomes apparent in mobile mapping applications such as road surface inspections: whereas cracks are apparent in lower-quality imagery, inside a LiDAR point cloud they’re harder to discover.
Interpreting Imagery versus Point Cloud Data
LiDAR, on the other hand, can detect certain types of objects better than photogrammetry, such as trees. Whereas plain point clouds show all points without any distinction between them, colored point clouds use point cloud classification. This is a pre-defined classification schema that colors each point inside a point cloud based on height, surface, or RGB values, among others. This makes the data easier to interpret and differentiate between different surface types.
The following example shows the subtle difference between LiDAR and photogrammetry:
LiDAR data is highly accurate, showing small variations in road surfaces very accurately. But it does not lend itself very well for visually interpreting such variations: this is where imagery would be a better option.
Combining LiDAR Point Clouds and Photogrammetric Imagery
To get the best of both worlds, it’s possible to capture both LiDAR and photogrammetric imagery at the same time and merge the results. Such data fusion is a solution for covering data gaps as a result of occlusion, which means objects blocking each other. It also makes up for missing data in either dataset, resulting in a more complete visual representation of the environment. Capturing both LiDAR and photogrammetric imagery of the same area also allows for the creation of a colored point cloud.
The Benefits of a Colorized Point Cloud from LiDAR and Photogrammetric Imagery
A colorized point cloud is a LiDAR point cloud with photo-realistic colors derived from camera-based imagery. This makes for easier visual interpretation, a more realistic user experience, and enhanced details, among others. Creating a colored point cloud is a challenging engineering process, but achieving a colored point cloud means that the underlying system is very accurately calibrated.
How a Colored Point Cloud is Created
This requires a photogrammetric camera to be synchronized with a LiDAR sensor to a very high level of accuracy. This means having images synchronized within microseconds, knowing how far the LiDAR sensor is from the camera, and very precise optical calibration of the lens to make sure the imagery pixels are distributed across the field of view. To get a colored point cloud, you use this information to decide where each camera pixel goes into the point cloud.
Performing Automated Object Detection
Capturing both LiDAR and photogrammetric imagery is not always an option. It’s also not required for deriving valuable information from raw point cloud data, such as object and surface types. We’ll now cover how you can extract valuable information through automated object detection in mobile mapping. This is an automated process that turns raw data into ready-to-use information. Also, it doesn’t require you to capture any LiDAR data: only photogrammetric imagery is enough.
Automated Object Detection in Mobile Mapping
To detect and classify different objects inside photogrammetric imagery, algorithms are used to detect faces and license plates and blur them so that these identifying features become anonymous. The opposite of this is ALPR, which is automated license plate recognition, for example for parking and speed enforcement. The same happens for street signs (road inventory management) and cracks in the road to monitor the state of the roads, as well as the curbs.
This process starts with human-annotated data, which is created by defining specific object types at the pixel level. This process requires coloring each pixel as exactly as possible. Next, after creating a few thousand images, these are fed into a neural network so it learns what the object is to be able to detect similar assets automatically. Better training data will result in better object recognition results.
How to Achieve Millimeter-accuracy with Photogrammetry
At the start of this article, we’ve argued that a positioning system is crucial for creating a good trajectory. We’ll now discuss how to achieve high-accuracy data for both LiDAR and photogrammetry, as accuracy is often one of the most important metrics used to compare both technologies. This will enable you to understand why with a bit of extra effort, photogrammetry can reach survey-grade accuracies similar to LiDAR.
Understanding Data Accuracy
Accuracy in photogrammetry and LiDAR is often expressed in relative and absolute accuracy. The terms horizontal and vertical accuracy are also used, which refer to the same principle: absolute (vertical) accuracy refers to how accurate a measured point is relative to its geographic position on Earth, while relative (horizontal) accuracy conveys the accuracy of the points within the same scene.
ABSOLUTE ACCURACY

RELATIVE ACCURACY

Photogrammetric Data Processing to Achieve Millimeter-accuracy
Because photogrammetry saw a later market adoption than LiDAR, laser scanning was often the first choice as it offered high-accuracy data with (high-end) out-of-the-box systems. Over time, the differences between LiDAR and photogrammetry have gotten smaller in terms of accuracy. Today, it’s possible to reach high accuracy (in millimeters) with photogrammetry if the data processing is done properly. Photogrammetry requires a substantial amount of processing time, especially when compared to LiDAR, but results in the same kind of global accuracy as LiDAR in general when done properly.
This includes creating correct ground control points that act as a ground truth for the boundaries of a dataset when processing the data later and enhance their spatial accuracy, and therefore, data quality. Coded targets are to be preferred over manually defined control points. The first is detected automatically while defining manual control points is a tedious task that is best avoided.
Mosaic’s Mobile Mapping Camera Systems
Mosaic offers a range of high-resolution, robust 360° cameras designed for mobile mapping and various other applications. Its product lineup includes several notable models, including the Mosaic 51, Mosaic X, and the Mosaic Viking. Mosaic’s products are widely used in applications such as land surveying, telecommunications, infrastructure maintenance, and post-disaster recovery.
Mosaic’s mapping devices use six image sensors: five circling the body of the camera, and one pointing up, which results in a full 360-degree view. The road is visible using an angle of 45 degrees downwards. The cameras capture up to 10 frames a second, which means one image every meter at a speed of 36 km/h. On highway inspections, driving 100 km/h results in a single image every three meters. When capturing imagery while driving, setting the shutter speed appropriately is important to avoid any motion blur.
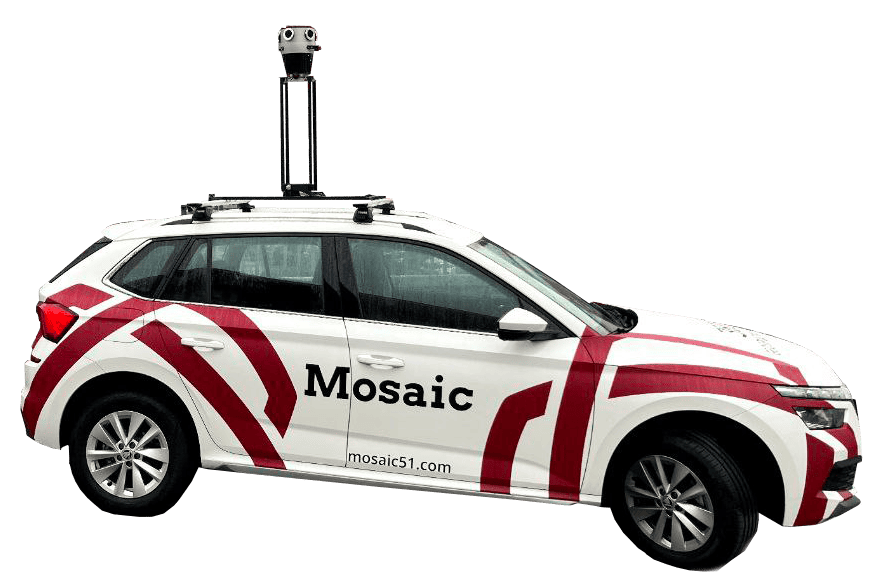
Driving Down Data Volumes
The cameras of Mosaic use image compression to drive down the data volumes. Another way to reduce the level of data to be consumed is by not delivering all data, but only the most relevant data. The more sensitive the data collection instruments are, the more data is collected which explains why data management is almost always going to be an issue. With very large datasets, data is processed both on the cloud and on a workstation. The cloud offers more flexibility to scale up or down when necessary.
Mosaic Xplor Backpack Solution
Mobile mapping started as camera-based systems mounted on cars and later trains, but today also includes backpack cameras so a person can walk around with a system mounted on a backpack. Mosaic recently launched a camera system in a backpack form factor, that also allows mapping indoor spaces. The Mosaic Xplor is a mobile mapping backpack equipped with a 14K resolution camera, LiDAR, and GNSS. Weighing just 7 kg, it’s designed for portability and can capture data at speeds up to 50 km/h.
A backpack mobile mapping solution is also a good solution for narrow roads unsuitable for cars. A driver with a backpack can drive a moped or the popular rentable scooters, available in most cities these days, on such a road. They are also used for any site that is accessible to pedestrians, and that requires high-frequency documentation, such as industrial facilities and construction sites.

challenging-to-reach environments – indoors and outdoors.
Conclusion
Choosing the right mobile mapping system is more than choosing between LiDAR or photogrammetry data acquisition. Before mobile mapping data is acquired, a trajectory needs to be defined for the mobile mapping to follow. Such a trajectory provides a basis for GIS-based inspection workflows, which can be further aligned using the mobile data acquired in the field.
Choosing the right mobile mapping system is crucial for accurate spatial data collection. Whether you opt for LiDAR, photogrammetry, or a combination of both, understanding their differences and applications will enhance your mapping projects.
Precise positioning technology is the secret ingredient for getting mobile mapping right and acquiring accurate spatial data outdoors. The value of a good mobile mapping trajectory is often overlooked when discussing data accuracy when acquiring mobile mapping, whether using LiDAR or photogrammetry.
LiDAR and photogrammetry are different technologies for capturing high-accuracy spatial data and producing 3D point clouds. When the different datasets are combined, a colored point cloud can be produced. To turn raw imagery data into useful information, automated object detection helps to recognize objects inside photogrammetric imagery.
Finally, photogrammetry can achieve millimeter-accuracy spatial data when the data is processed in the right way. Mosaic’s high-resolution, robust 360° cameras are designed for mobile mapping and various other applications. They have been praised for their high-resolution imagery, durability, and integration capabilities with various software and systems.
Explore our advanced camera-based systems to achieve the highest accuracy in your mobile mapping endeavors.
FAQ
Q: Can you create point clouds from both photogrammetry and LiDAR?
A: Yes, you can create point clouds from both photogrammetry and LiDAR. However, a LiDAR scanner creates and stores 3D points in a point cloud directly, whereas, with photogrammetry, cameras create large sets of images. These images first need to be processed using photogrammetry software, after which a 3D point cloud can be created.
Q: Where is the market for photogrammetry and LiDAR heading?
A: LiDAR and photogrammetry systems are continuously improving, and new sensors and systems are entering the market. This is leading to a convergence of systems, as the difference between LiDAR and photogrammetry is getting smaller in terms of accuracy, acquisition time, and possible use cases.
Q: Should I invest in LiDAR or photogrammetry?
A: This depends on multiple factors: budget, the required data and deliverables, the application, what you want to capture, and how fast you need to operate, among others. Therefore, it’s impossible to say which technology best meets your needs. However, unless you’re addressing specific use cases where LiDAR makes the difference, a camera-based system will meet your data accuracy requirements, and also save you a lot of money. LiDAR shines in use cases such as large-scale mapping, vegetation mapping, and corridor mapping. These use cases all require airborne or drone-based LiDAR, but for mobile mapping, millimeter-accuracies can be achieved that are similar to LiDAR if necessary. For inspection-based mapping, such accuracies are not necessary.
Q: Can LiDAR penetrate solid surfaces?
A: No, LiDAR cannot solid surfaces. It would be better to compare LiDAR with how light works, for example, if you are underneath dense vegetation and see light coming in from above through the vegetation, the same will happen if you map that vegetation from above: LiDAR pulses will be able to penetrate through the holes between the leaves, just as light enters.